Everything You Should Know About Data-Science
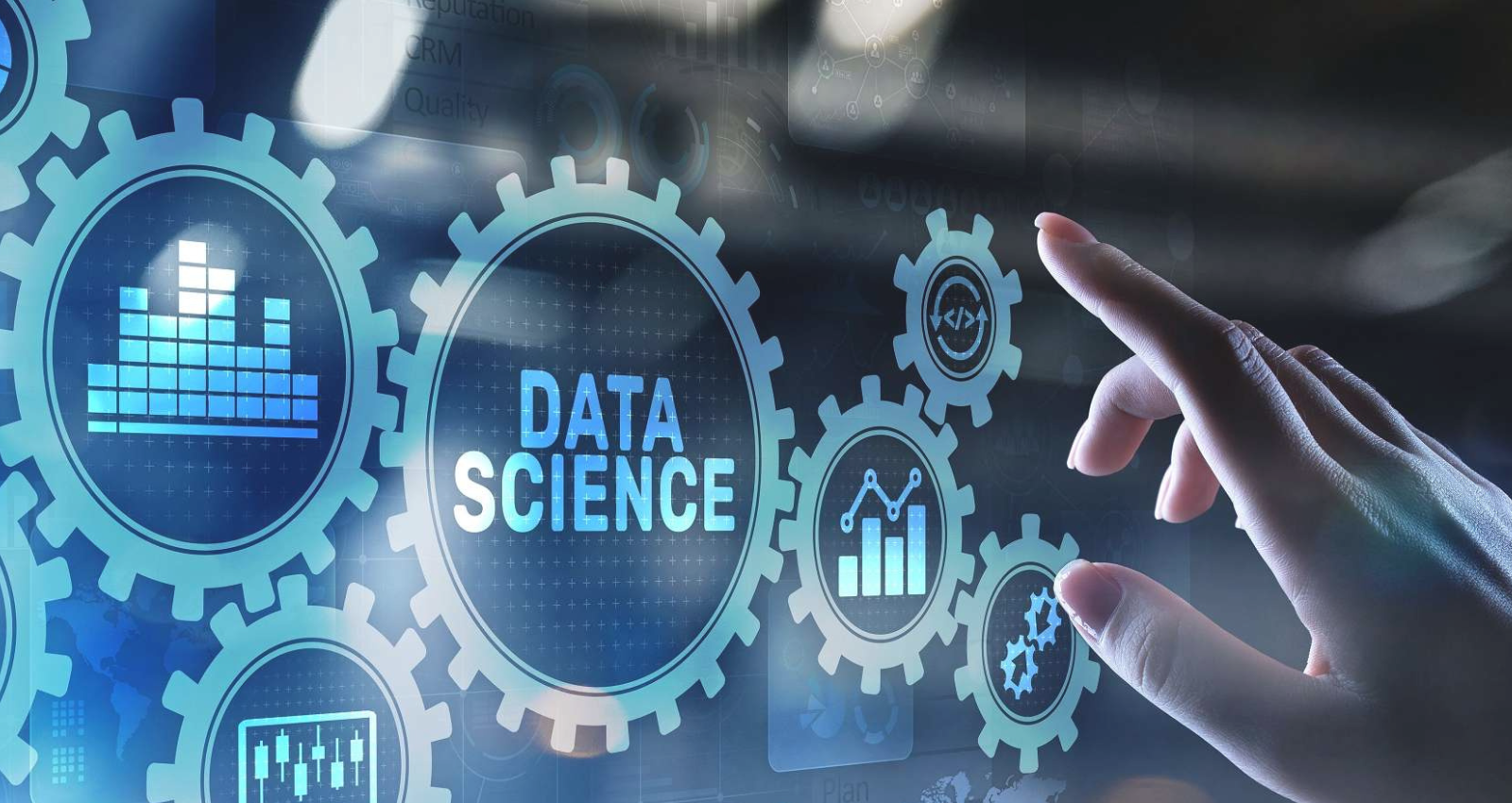
What is Data Science?
According to a groundbreaking study published in 2013, 90 percent of the world’s data was created in the previous two years. Allow that to sink in. We’ve collected and processed 9x the amount of data in the last two years than the previous 92,000 years of humankind combined. And it shows no signs of slowing down. It’s estimated that we’ve already generated 2.7 zettabytes of data, with that figure expected to skyrocket to 44 zettabytes by 2020.
Our digital data is widely regarded as the “oil of the twenty-first century,” and it is the most valuable resource in the field. It has incalculable advantages in business, research, and our daily lives. Your commute to work, your most recent Google search for the nearest coffee shop, your Instagram post about what you ate, and even your fitness tracker’s health data are all important to different data scientists in different ways. Data science is responsible for bringing us new products, delivering breakthrough insights, and making our lives more convenient by sifting through massive lakes of data in search of connections and patterns.
How Data Science works?
Data science entails a wide range of disciplines and expertise areas to produce a comprehensive, thorough, and refined examination of raw data. To effectively sift through muddled masses of information and communicate only the most vital bits that will help drive innovation and efficiency, data scientists must be skilled in everything from data engineering, math, statistics, advanced computing, and visualizations.
Data scientists also heavily rely on artificial intelligence, particularly its subfields of machine learning and deep learning, to create models and predict outcomes using algorithms and other techniques.
Recognize the business issue:
The data science process begins with understanding the problem that the business user is attempting to solve. For example, a business user may wish to inquire and comprehend “How do I increase sales?” or “What methods work best for selling to my customers?” These are broad, ambiguous questions that do not immediately lead to a researchable hypothesis.
Collect and integrate raw data:
Once the business problem has been identified, the next step is to collect and integrate the raw data. The analyst must first determine what data is available. Data is frequently in a variety of formats and systems, so data wrangling and data prepping techniques are frequently used to convert raw data into a usable format suitable for the specific analytic techniques that will be used. If the data isn’t available, data scientists, data engineers, and IT usually work together to get new data into a sandbox environment for testing.
Data exploration, transformation, cleaning, and preparation:
The data can now be explored. Most data science practitioners will use a data visualization tool to organize the data into graphs and visualizations that will allow them to see general patterns in the data, high-level correlations, and potential outliers. This is also the point at which the analyst begins to understand which factors may aid in the resolution of the problem. Now that the analyst has a basic understanding of how the data behaves and potential factors to consider, the analyst will transform the data, create new features (aka variables), and prepare it for modeling.
Create and choose models based on data:
The data can now be explored. Most data science practitioners will use a data visualization tool to organize the data into graphs and visualizations that will allow them to see general patterns in the data, high-level correlations, and potential outliers. This is also the point at which the analyst begins to understand which factors may aid in the resolution of the problem. Now that the analyst has a basic understanding of how the data behaves and potential factors to consider, the analyst will transform the data, create new features (aka variables), and prepare it for modeling.
Models are tested, tuned, and deployed:
To test different models, most analysts will use algorithms to create models from the input data using techniques such as machine learning, deep learning, forecasting, or natural language processing (aka text analytics). Statistical models and algorithms are applied to the dataset in an attempt to generalize the behavior of the target variable (for example, what you’re attempting to predict) based on the input predictors (for example, factors that influence the target).
Models must be monitored, tested, refreshed, and governed:
After the models are deployed, they must be monitored so that they can be refreshed and retrained as data shifts due to changing real-world event behavior. As a result, organizations must have a model operations strategy in place to govern and manage changes to production models.
Data scientists may create sophisticated data science pipelines that can be invoked from a visualization or dashboard tool in addition to deploying models to dashboards and production systems. These frequently have a reduced and simplified set of parameters and factors that a citizen data scientist can adjust. This contributes to addressing the skills shortage. As a result, a citizen data scientist, who is frequently a business or domain expert, can select the parameters of interest and run a very complex data science workflow without having to understand the complexities involved.
Uses of Data Science:
Here are a few examples of how companies are using data science to innovate in their industries, create new products, and make the world around them more efficient.
Health Care:
In the healthcare industry, data science has resulted in several breakthroughs. With a vast network of data now available through everything from EMRs to clinical databases to personal fitness trackers, medical professionals are discovering new ways to understand disease, practice preventive medicine, diagnose diseases more quickly, and investigate new treatment options.
Self–driving cars:
Data science has resulted in many breakthroughs in the healthcare industry. Medical professionals are discovering new ways to understand disease, practice preventive medicine, diagnose diseases more quickly, and investigate new treatment options thanks to a vast network of data now available through everything from EMRs to clinical databases to personal fitness trackers.
Logistics:
UPS uses data science to boost efficiency both internally and along its delivery routes. The company’s On-road Integrated Optimization and Navigation (ORION) tool employs data science-backed statistical modeling and algorithms to generate optimal routes for delivery drivers based on factors such as weather, traffic, and construction. Each year, data science is estimated to save the logistics company up to 39 million gallons of fuel and more than 100 million delivery miles.
Finance:
The banking industry has saved millions of dollars and untold hours thanks to machine learning and data science. For instance, the Contract Intelligence (COiN) platform from JP Morgan uses Natural Language Processing to process and extract vital information from over 12,000 commercial credit agreements each year (NLP). Thanks to data science, what would have required over 360,000 hours of human labor may now be finished in a matter of hours. Furthermore, to create machine learning technologies that identify and stop fraud, fintech firms like Stripe and Paypal are making significant investments in data science.
Cybersecurity:
Data science is beneficial in all industries but maybe most important in cybersecurity. Kaspersky Lab, an international cybersecurity firm, detects over 360,000 new samples of malware every day using data science and machine learning. Data science will allow us to detect and learn about emerging cybercrime techniques in real-time, which is essential for our safety and security in the future.
Entertainment:
Do you ever wonder how Spotify seems to know exactly what song you’re looking for? Or how does Netflix know which shows you’ll enjoy binge-watching? The music streaming service can carefully curate lists of songs based on the music genre or band you’re currently into using data science. Are you getting into cooking lately? Netflix’s data aggregator will recognize your desire for culinary inspiration and recommend relevant shows from its vast library.
In business, data and data specialists are immensely valuable. If anybody is interested in a job in data science, they should take into account the fact that critical thinking and machine learning algorithms can provide insights for making crucial business decisions.