Potential of Generative AI for Enterprise Analytics
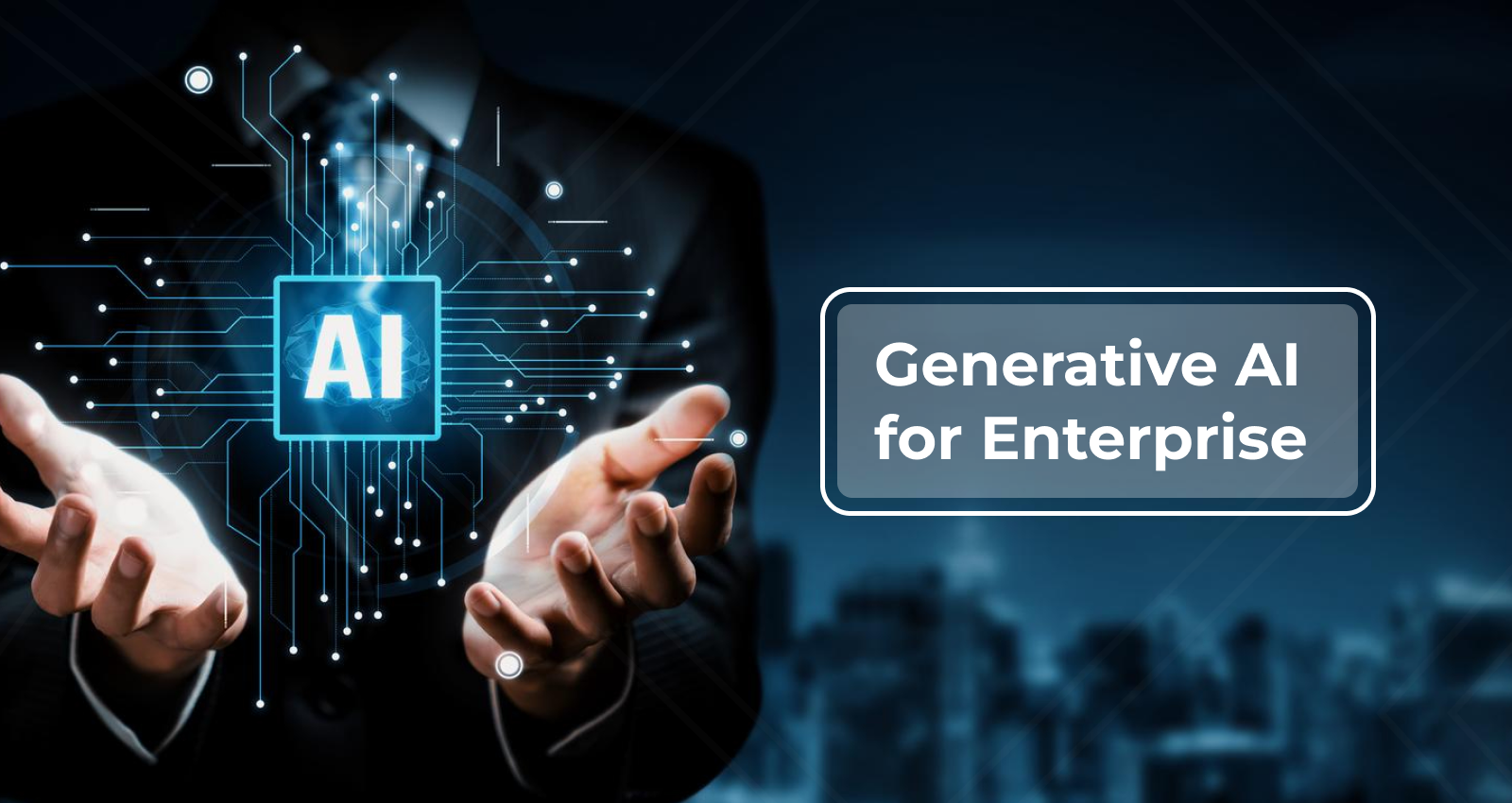
Introduction
In today’s fast-paced business environment, data is more than just numbers. It’s the key to making smart decisions and staying ahead of the competition. Generative AI, a subset of artificial intelligence that can create new content and patterns from existing data, is transforming enterprise analytics. By using generative AI, companies can unlock deeper insights, predict trends, and drive innovation. This blog explores how generative AI can revolutionize enterprise analytics, the challenges businesses face, and strategies to overcome these hurdles.
The Current Landscape of Generative AI Adoption
Generative AI is becoming a game-changer in enterprise analytics. Many companies are already using it to enhance their data analysis and decision-making processes. Let’s look at two case studies to understand its impact better.
Case Study 1: Retail Industry
A leading retail company used generative AI to analyze customer purchase patterns. By integrating AI into their analytics, they could predict future buying trends and personalize marketing campaigns. This resulted in a 20% increase in sales and a 15% improvement in customer retention. The AI model could analyze vast amounts of data from different sources, such as social media, purchase history, and online behavior, providing a holistic view of customer preferences.
Case Study 2: Healthcare Sector
In healthcare, a major hospital implemented generative AI to predict patient readmissions. By analyzing patient data, including medical history, treatment plans, and recovery patterns, the AI could identify high-risk patients. This proactive approach reduced readmissions by 30% and improved patient care. The AI system also helped in optimizing resource allocation, ensuring that high-risk patients received the necessary attention and treatment on time.
These case studies highlight the potential of generative AI in transforming how businesses analyze and utilize their data. However, the journey is not without its challenges.
Pinpointing the Barriers
Despite its potential, several barriers hinder the full adoption of generative AI in enterprise analytics. These barriers can be broadly categorized into people, systems, and data.
1. People: One of the significant barriers to the adoption of generative AI in enterprise analytics is the human element. Many organizations face a shortage of skilled professionals who can understand, implement, and manage AI technologies. Additionally, there is often resistance to change among employees who fear job loss or disruption to their established work routines. Overcoming these challenges requires comprehensive training programs to bridge the skill gap and effective change management strategies to ensure a smooth transition and acceptance of AI technologies within the workforce.
Skill Gap: Many organizations lack skilled professionals who can understand and implement generative AI. This skill gap can slow down the adoption process.
Resistance to Change: Employees may resist new technologies due to fear of job loss or disruption in their work processes. Overcoming this resistance requires proper change management strategies.
2. Systems: Legacy systems pose a significant barrier to integrating generative AI in enterprise analytics. Many organizations still rely on outdated IT infrastructure that is incompatible with advanced AI technologies. Upgrading or replacing these systems to support AI can be both costly and time-consuming. Additionally, as data volume grows, existing systems may struggle with scalability, which can hinder the performance and efficiency of AI models. Addressing these issues involves significant investment in modernizing IT infrastructure, ensuring that it can handle the increased data load and support the seamless integration of AI capabilities.
Legacy Systems: Older systems may not support the integration of advanced AI technologies. Upgrading or replacing these systems can be costly and time-consuming.
Scalability Issues: As the amount of data grows, the existing systems may struggle to scale up to handle the increased load, affecting the efficiency of AI models.
3. Data: Data-related challenges significantly impact the adoption of generative AI in enterprise analytics. Poor-quality data can lead to inaccurate predictions and unreliable insights, undermining the effectiveness of AI models. Moreover, data is often stored in silos across different departments, making it difficult to integrate and analyze comprehensively. This fragmentation hampers the AI’s ability to provide holistic and accurate insights. Ensuring high data quality through regular audits, standardization, and cleaning processes is essential. Additionally, breaking down data silos and facilitating seamless data integration across the organization is crucial for maximizing the potential of generative AI.
Data Quality: Poor-quality data can lead to inaccurate predictions and insights. Ensuring data accuracy and consistency is crucial for effective AI implementation.
Data Silos: Data stored in different silos across the organization can be challenging to integrate. This fragmentation hampers the AI’s ability to provide comprehensive insights.
Leveraging Generative AI for Added Efficacy
To fully leverage generative AI, businesses need to adopt a strategic approach that addresses the barriers and maximizes the benefits of AI technology.
Building a Skilled Workforce: Investing in training and development programs for employees is essential. Organizations should focus on upskilling their workforce with AI and data analytics skills. Partnerships with educational institutions and online learning platforms can help in creating a continuous learning environment.
Upgrading Systems and Infrastructure: Modernizing IT infrastructure to support AI integration is critical. This includes upgrading legacy systems, enhancing data storage capabilities, and ensuring scalability. Cloud-based solutions can provide the necessary flexibility and scalability required for AI operations.
Ensuring Data Quality and Integration: Implementing robust data governance practices ensures high data quality. Regular data audits, standardization, and cleaning processes can help maintain data integrity. Additionally, using data integration tools can break down silos and facilitate seamless data flow across the organization.
Balancing Generative AI with a Unified Analytics Approach
While generative AI offers numerous advantages, it should not operate in isolation. Integrating it with a unified analytics approach ensures that businesses can extract maximum value from their data.
Combining Traditional and AI-Driven Analytics: Traditional analytics methods provide valuable insights but combining them with AI-driven analytics can enhance the depth and accuracy of the analysis. A hybrid approach leverages the strengths of both methods, leading to more informed decision-making.
Creating a Centralized Data Repository: Establishing a centralized data repository where all data is stored and accessible can improve the effectiveness of AI models. This repository should be equipped with advanced analytics tools and governed by strict data management policies.
Fostering a Data-Driven Culture: Promoting a data-driven culture within the organization is essential. This involves encouraging employees at all levels to rely on data for decision-making and providing them with the necessary tools and training to analyze data effectively.
Conclusion
Generative AI holds immense potential to revolutionize enterprise analytics, offering deeper insights, enhanced predictions, and innovative solutions. However, to unlock its full potential, businesses must address barriers related to people, systems, and data. By building a skilled workforce, upgrading systems, ensuring data quality, and integrating AI with a unified analytics approach, organizations can harness the power of generative AI to drive growth and stay competitive.
Embracing generative AI is not just about adopting new technology; it’s about transforming the way businesses operate and make decisions. With the right strategies and a commitment to continuous improvement, enterprises can fully realize the benefits of generative AI in their analytics journey.
Are you looking for any IT Services such as Software Testing, Web design and Development, Professional/Staff Augmentation Services, Cloud Computing, Mobile App Development, Digital Marketing Services and more? Connect Stridefuture Technology, which helps to meet your requirements.
{StrideFuture Technology, a full-service company specializing in Software Solutions and Consultancy services. We specialize in Personal, Business, IT Services, Software Testing, Web design and Development, Mobile App Development, Digital Marketing Services, and much more you can dream Virtually with us! Reach out for more service at StrideFuture Technology.}